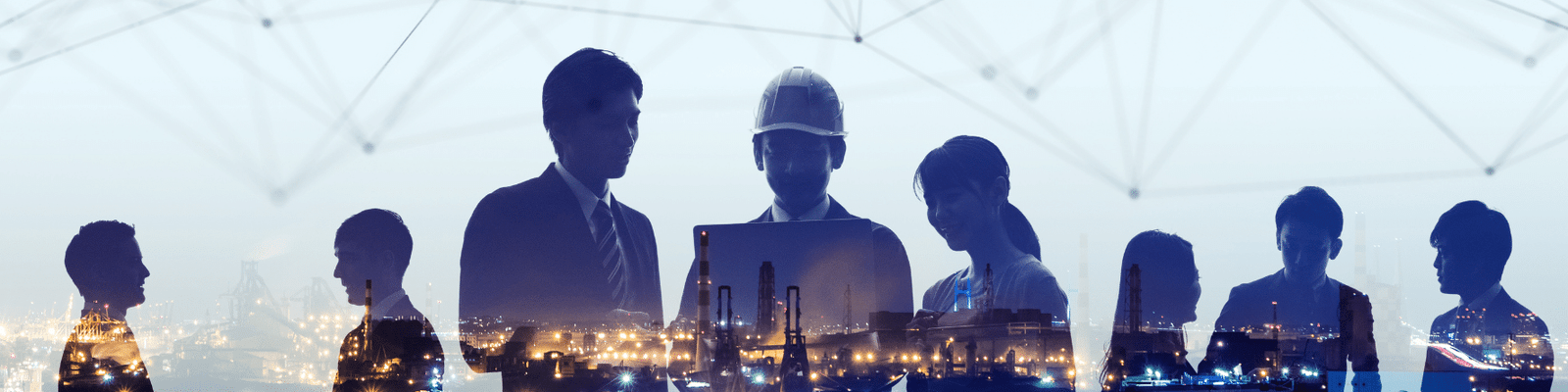
Predictive Analytics in HR
It’s safe to say that remote work has brought a previously unthinkable level of digitization into all corporate processes and interactions. As a result, HR managers have ended up with an unexpected amount of data on their hands and have had to seek predictive analytics expertise to make grounded and proactive decisions based on it.
Here, we’ll take a look at a few of the key areas where predictive analytics software can benefit human resources operations in an organization, from candidate procurement to human network and employee churn analysis. But before that, let’s figure out what predictive analytics in HR means and discuss its benefits.
What is predictive analytics in HR?
Predictive analytics in HR is using data mining, statistics, and machine learning to make more informed decisions about recruiting, staffing, and other HR functions. Whether it’s identifying employees that are at risk of quitting or determining the best candidate for a position, by analyzing data from CVs, job descriptions, and HRIS systems, predictive analytics can significantly improve talent management.
Predictive analytics in HR market stats
10 benefits of predictive analytics in HR
1 | Improved recruiting | With the help of machine learning, companies can determine what qualities are the most important for a particular role and accurately predict which candidates will better fit the organization both culturally and skill-wise. |
2 | More accurate performance evaluation | Predictive analytics allows companies to get a deeper insight into employees’ efficiency of work, which can help companies improve talent management. |
3 | Decreased turnover rates | With predictive analytics, HR professionals can spot employees who are on the verge of quitting and timely prevent their departure, ultimately decreasing turnover rates. |
4 | Increased employee engagement | By having a more granular understanding of employees’ behavior, HR departments can optimize employee onboarding, enhance team collaboration, and take actions to improve employee performance overall. |
5 | Improved decision-making | With the help of predictive analytics, computer vision, machine learning, and NLP, video-based candidate interview assessments can be partially automated and the process itself significantly improved. |
6 | Enhanced talent discovery | Machine learning models applied in HR can provide deeper insight into employees’ performance and skills, which can help organizations to spot candidates for management positions. |
7 | Improved employee upskilling | Predictive analytics-based HR platforms can detect employees’ skill gaps, enabling companies to make evidence-based decisions about workforce upskilling. |
8 | Reduced hiring costs | Given that predictive analytics models can automatically process enormous amounts of employee data, companies can allocate fewer human resources to these manual tasks. |
9 | Improved communication | NLP-based software can help HR departments to understand which communication and linguistic patterns are the most engaging, motivational, and appealing for candidates or existing employees. |
10 | Decreased hiring bias | Carefully tuned and well-thought-out predictive models can minimize hiring bias and make hiring more inclusive in terms of race, gender, age, nationality, etc. |
1. Candidate sourcing and filtering
When casting an initial net for possible job candidates, AI will almost certainly be involved in the process. Several machine learning sectors have been brought to bear on this sector of HR, including natural language processing (NLP), computer vision, audio analysis, and expression recognition. All of the largest recruitment consultants have availed themselves to some extent of HR software development in one or more of these sectors.
2. Head-hunting
Traditionally, candidates have been sourced either by arbitrary responses to job ads or via agencies and employment platforms to which candidates have subscribed. However, AI-based analytics, along with RPA in HR, offers more proactive methods for head-hunting prospective employees, so-called passive candidates, ‘in the wild’.
Predictive analytics tools and techniques in HR are capable of scouring the web for individuals that are suited to your organization, with a growing number of companies offering AI-based ‘broad sweep’ candidate retrieval. Such companies not only identify possible candidates from a variety of online sources but also calculate how receptive the person might be to a job offer and what salary level, perks, and other benefits can bring them into the fold.
For larger companies that are either growing or have higher turnover rates and that require such services on a more regular basis, it’s possible to develop in-house AI systems that operate on the same principles. However, it should be considered that the legal status of web scraping remains in a volatile state, with many of the most fruitful domains blocking or impeding systematic access to all ‘bots’ except those of the major search engine providers.
3. Social profile analysis
The extent of the data that’s being considered in employee applications is growing: the recruiter platform Arya scours the internet looking for social network and membership profiles associated with a candidate, seeking potential ‘red flags’ such as ‘extreme political views‘, drink- or drugs-related content, disclosure of confidential information about previous employers, discriminatory comments, and many other possible red flags. The system can also make predictions about the likelihood of an employee leaving the company (see ‘Predicting employee churn’ below).
As with automated domain-scraping candidate search systems, live or systematic analysis of social media platforms are subject to site terms and, frequently, protections from the systematic polling that powers them, which may place some restrictions on automated profile analysis both for third-party providers and for the development of in-house systems.
4. Effective communication with NLP
In a nutshell, the majority of HR processes rely on some sort of text-based communication, which NLP-enabled systems can quickly analyze at scale.
Surveys and questionnaires, a longtime staple of multi-stage candidate assessment, can now be incorporated into NLP systems that can provide a more interactive and responsive study of a candidate, for instance through chatbots.
Whether it’s a company-wide announcement via email, or private dialog with a particular employee, by making informed language style choices down to a single phrase and word, HR professionals can craft messages that are more motivating and engaging for employees.
VoIP-based interview video footage can be analyzed not only in terms of emotion recognition and image-based evaluation but also parsed into text, and the text analyzed by NLP systems. Recruiters can use NLP to better classify candidate profiles and minimize bias. Unlike humans, predictive analytics systems make insights based solely on qualitative data, rather than on gut feeling and industry experience.
5. Job candidates’ facial analysis
The most enduring long-term strengths for HR predictive analytics at the interview stage are proving to be based on what the candidate says at interview time through automated data collection and analysis of auto-transcribed text from video and audio recording sources.
Though the COVID-driven move from face-to-face interviews over to VoIP-based procedures has made applying facial analysis far easier, face-based emotion recognition techniques remain controversial, and their deployment should be carefully considered from the standpoints of legality and efficiency.
For example, HireVue initially included facial analysis and emotion recognition in its candidate evaluation software but announced early in 2021 that it would remove this functionality from its offerings following the results of an algorithm audit. The company’s chief data scientist maintains that in most cases non-verbal data contributes no more than 0.25% of a model’s predictive capabilities.
Following the announcement that IBM, Microsoft, and Amazon would discontinue various implementations of facial recognition and analysis in response to protest over algorithmic racial discrimination, the Co-Director of the AI Now Institute, Meredith Whittaker, compared the use of facial analysis in candidate review to the long-discredited study of phrenology (discerning personality through a person’s head-shape), and that systems based around it lack a basis of solid scientific consensus.
Still, facial analysis remains a work in progress, underpinned by various highly-contested theories, and may prove to be a more useful tool in later years, after further development and consolidation, rather than in this period.
6. Meeting analysis
Besides the use of predictive analytics for voice interviews, for instance by analyzing auto-transcription of VoIP-based candidate interviews, it’s possible to use machine learning to uncover insights from meetings, whether they took place in the real world or online.
However, it’s worth repeating the earlier-mentioned warning around face-based analytics systems — all participants should ideally be enthusiastically participating in such systems. Also, under a growing tranche of regulations around automated monitoring, it will usually be necessary to disclose their use in advance.
7. Employee monitoring
HR predictive analytics can provide a wide range of insights into the way employees are achieving their performance goals and the company’s objectives. For example, ecommerce enterprises may use transaction and sales data collected by their POS systems to determine the most productive employees and the best sales channels.
Simple machine learning methods, such as K-Nearest Neighbors, are highly applicable to performance analysis and prediction. K-Means Clustering and Decision Trees, which are two of the oldest methods of machine learning analysis, can also be used to devise predictive analytics systems capable of ongoing performance evaluation.
Real-life examples of predictive analytics in HR
LinkedIn Recruiter
The immense advances of LinkedIn’s machine learning research are unsurprising since the company has one of the richest datasets in the world that grows exponentially day by day.
LinkedIn Recruiter helps recruiters and hiring managers to streamline talent sourcing and improve hiring outcomes overall. When a recruiter makes a candidate search request, a predictive analytics system ranks candidates that match a specific request based on their work experience, skills, job posting time and location, etc.
LinkedIn Recruiter uses Gradient Boosted Decision Trees (GBDTs), among many other intensive machine learning approaches, to calculate ‘non-obvious’ factors that may align a potential recruit with the interests of an employer.
HireVue
The Utah-based HR tech company HireVue performs text analysis on candidate interviews, combined with various other signifiers of suitability developed by the company’s psychological research teams.
The company uses proprietary machine learning models that analyze video-based interviews to predict the candidate’s future job performance. Most interestingly, the platform can assess non-verbal cues like facial expressions, eye movements, body language, and even personal style to enable companies much deeper insight into a candidate’s personality than any other form of an interview. The effectiveness of this AI-powered system can be proven by the caliber of its clients: Deloitte, Goldman Sachs, Amazon, and Unilever.
Textio
Textio is the augmented writing platform that uses predictive analytics and NLP to help HR professionals to craft messages that resonate the most with both existing employees and potential ones.
For example, recruiters use Textio to reveal business jargon, age, and gender bias phrases that turn off candidates, and receive suggestions on what language will appeal the most to a certain audience. Importantly, Textio augments writing based on the language stack that a company already uses, allowing organizations to stay consistent in terms of style and branding across all communication channels.
Remesh
Remesh is an audience intelligence platform that allows enterprises to better understand their audiences, be they employees or consumers. With their predictive analytics-powered platform, Remesh helps companies to capture authentic employee feedback, which can be used to make data-backed decisions about employee health and benefits management, change management, diversity and inclusion, and cultural transformation.
To employees, Remesh’s platform looks like a combination of a simple messenger app and a survey platform, where an HR professional asks both closed- and open-ended questions. Remesh analyzes employees’ responses in real time, segments them into groups, and provides actionable insights. Given that the platform provides anonymity to the employees’ responses, there is a high probability of authentic answers.
Headroom
The AI meeting analysis framework Headroom, which has recently raised $9 million, can automate note-taking and uses emotion recognition algorithms to determine what impression a presentation is making on the ‘silent’ listeners in the room, providing the speaker with real-time feedback in a console window of their screen. The system draws on many factors, including pupil dilation, eyebrow disposition, mouth shape, and other groups of facial landmarks.
HR predictive analytics implementation advice
Don’t jump on the hype train
The pandemic has notably accelerated the adoption of HR predictive analytics systems and driven digital transformation in HR. While this may be the most promising time ever to implement reliable and established machine learning algorithms into your own HR department’s forecasting systems, it’s important to have a clear understanding of what the company is trying to achieve, and how success or failure can be quantified in non-ambiguous ways that lend themselves well to analytical systems. This is not a problem that machine learning can solve for you.
Prepare data
It’s necessary to establish whether the means to generate data that will drive your predictive analytics system currently exist. Though it can be tempting to leverage historical in-house data simply because there is already years’ worth of reports and logs to analyze, it may be better to start fresh by building more suitable data architectures that will begin to bear fruit in a year or so. Also, pay attention to the necessary equipment and methodologies that will constitute a value-driven business intelligence pipeline.
Determine the metrics
HR predictive analytics systems should also contain relevant metrics. Beyond fundamental aspects such as attendance, sick leave and holiday planning coordination, HR predictive analytics adopters should develop meaningful benchmarks of success that reflect a positive impact on the company.
These metrics can be difficult to establish since attendance and good productivity are not inextricably linked or participation in surveillance platforms (such as Slack or an internal company channel) does not automatically mean that useful and/or timely work is being done. In fact, it could well mean the opposite.
Certain industries have intrinsic metrics (such as social media shares obtained in PR organizations, traffic fluctuations in SEO operations, or units produced in industrial settings) that can be input into an employee’s predictive analytics profile. But where productivity is more subjective, companies would need to make an effort to implement accurate metrics.
Choose ML models wisely
It’s not always necessary to expensively train a large curated corpus of data in order to run machine learning-based predictive analytics on internal company data. So long as your dataset is consistent and old enough to yield meaningful year-on-year statistical trends, many of the ‘lighter’ approaches will suit the majority of companies.
In fact, a research initiative studied a number of ML approaches, some more resource-intensive than others, and ultimately favored the K-Nearest Neighbors (KNN) algorithm. KNN was formulated by the US military in 1951 and is known as ‘the lazy learner’ since it traverses the entire dataset for a ‘nearest neighbor’ prediction, instead of needing to actually train a machine learning model to make predictions based on historical data.

KNN is efficient and often features as a component even in more sophisticated and expensive analytics systems — and it may be all you need to get the job done. However, since KNN trawls through every single data point in search of analytical trends, it’s unsuitable for complex, high-volume data, such as the thirty-year history of a major global company (though this challenge can be addressed by Principal Component Analysis, an algorithm that can ‘slim down’ high-volume datasets into broadly representative, much lighter sets).
Other lower-impact approaches can be developed by similarly ‘established’ machine learning analysis techniques. In 2021, the Academy of Entrepreneurship Journal published a study into an ML-based employee retention framework using basic components such as Support Vector Machine and Random Forest.
Using this method, the authors were able to establish retention probabilities based on various interesting factors, such as academic qualifications, contract type, department placement, and even the employee’s degree major and degree level.
Implementation challenges of predictive analytics in HR
Talent shortage | When trying to implement predictive analytics, the majority of HR departments in companies face a lack of skilled talent.While most applications of predictive analytics in HR are user-friendly, the majority of them call for substantial data literacy, which is a rarity among HR professionals. | Unless you have a grandiose multi-year analytics program in place, we would highly suggest upskilling the current workforce rather than hiring data scientists, ML engineers, etc. When it comes to realizing the full potential of predictive analytics in HR, the specific knowledge and experience of HR professionals are as invaluable as the field expertise of data scientists. |
Insufficient IT resources | Running predictive analytics and data analytics in HR is a very demanding process from the IT perspective. Smaller companies with poor cloud infrastructures will have the hardest time implementing these analytics programs at scale. | Turning to the established SaaS platform for cloud resources is the most effective method for tackling this problem. |
Regulatory compliance and privacy | Predictive analytics can raise a number of regulatory challenges regarding data privacy, bias, and employee monitoring. | From a legal standpoint, it’s essential to stay on top of the turbulent regulatory environment surrounding employees’ rights regarding workplace monitoring and AI systems and, if necessary, to take professional advice about protecting the company from legal exposure. |
ROI and cost aspects
- 93% of talent-optimized companies were able to avoid major financial hardship caused by COVID-19. (Predictive Index)
- Companies with the right people in the right roles have 42% lower turnover. (Predictive Index)
- The average budget per user for HRIS software is $12,625 or approximately $210 per user per month. (Software Path)
- 42% of companies report that the profitability of AI projects exceeded their expectations. (Accenture)
- Companies that buy off-the-shelf AI solutions for HR can expect ROI within the first three to six months. (IBM)
- IBM realized $107 million in savings as a result of AI for HR adoption. (IBM)
Closing thoughts
With all its regulatory nuances and ethical implications, predictive analytics solutions is a great addition to the digital workplace toolkit of recruiters and talent managers. With an abundance of historical data, enhanced data governance practices, and continuously enlarging datasets, machine learning-based forecasting is becoming more accurate than ever before. Complex regulatory space around predictive analytics in HR shouldn’t scare off organizations, but make them more cautious and intentional about their AI adoption.